Accurate Indoor Maps with Affordable Sensors
- Shaoshan Liu
- May 8, 2019
- 2 min read
This is part of the PerceptIn technology blog on how to build your own autonomous vehicles and robots. The other technical articles on these topics can be found at https://www.perceptin.io/blog.
In this short passage we describe PerceptIn’s latest technology of using highly affordable sonar and other sensors (such as LiDAR or computer vision) to generate an accurate indoor map for robot navigation, this technology brings three advantages brought by our latest technology:
Chassis agnostic: we have tested this technology on multiple chassis with different sonar and other sensor configurations, and it worked in all cases.
Sonar only mapping: We also confirm that it is possible to use only sonar for mapping, given that other sensors (such as vision or LiDAR) provide accurate real-time locations. If we added LiDAR map to Sonar map we get a more accurate map. The combination of sonar and LiDAR enables the robot to smoothly navigate through the environment even when LiDAR fails to work.
Glass proof: unlike LiDAR, sonar map is glass proof thus effectively detect any glass or transparent obstacles.
First, we built the following small robot, which consists of a very cheap LiDAR device and 16 sonar sensors, the total sensor cost is less than $100 USD. We performed temporal and spatial calibration and utilized the robot for indoor map collection.
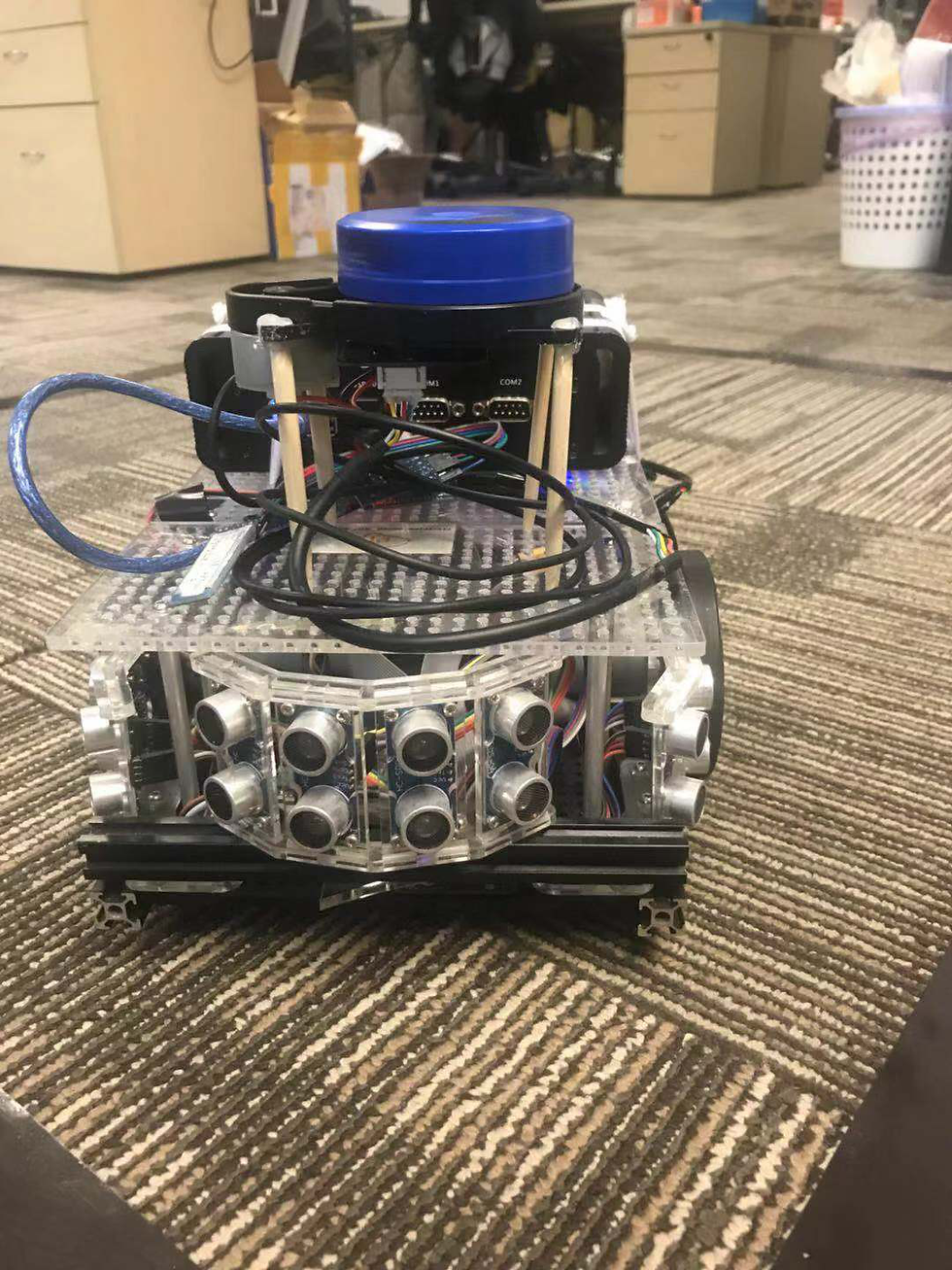
To make sure we tested it in a suitable environment, we chose the corridor outside of our office (Figure 2, Figure 3, Figure 4), which contains many glass doors that LiDAR will penetrate, and LiDAR alone would not be able to generate a good map.
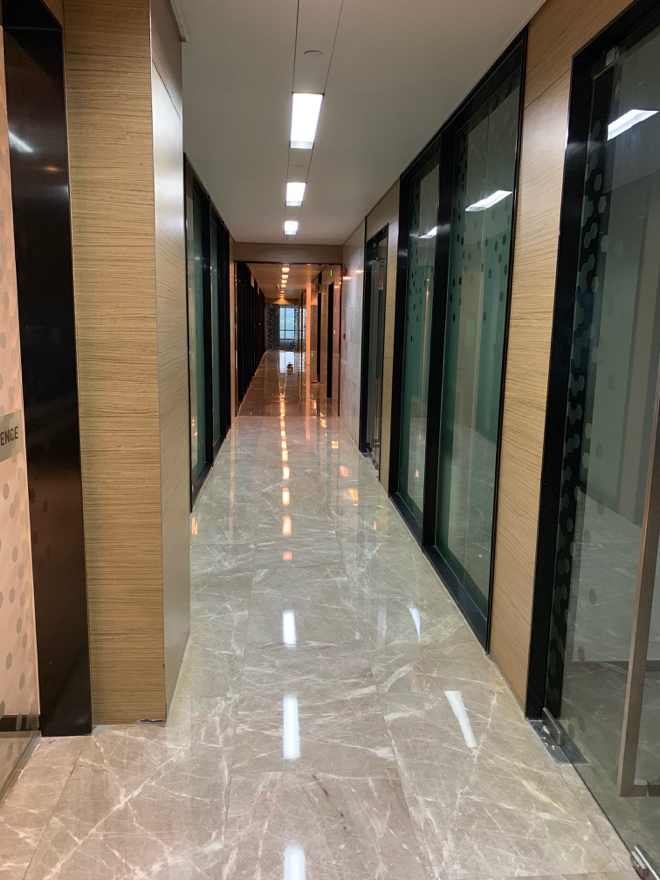
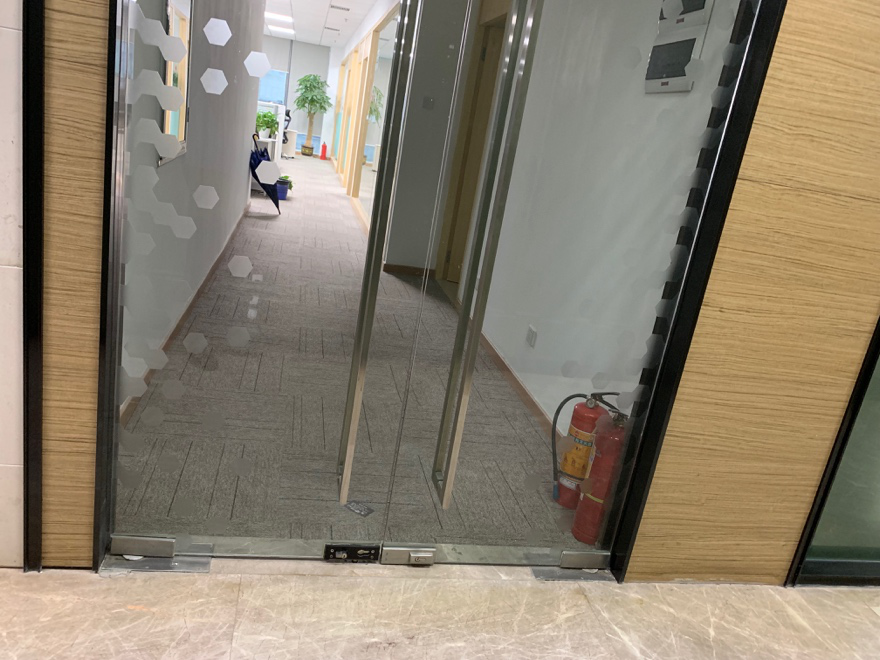
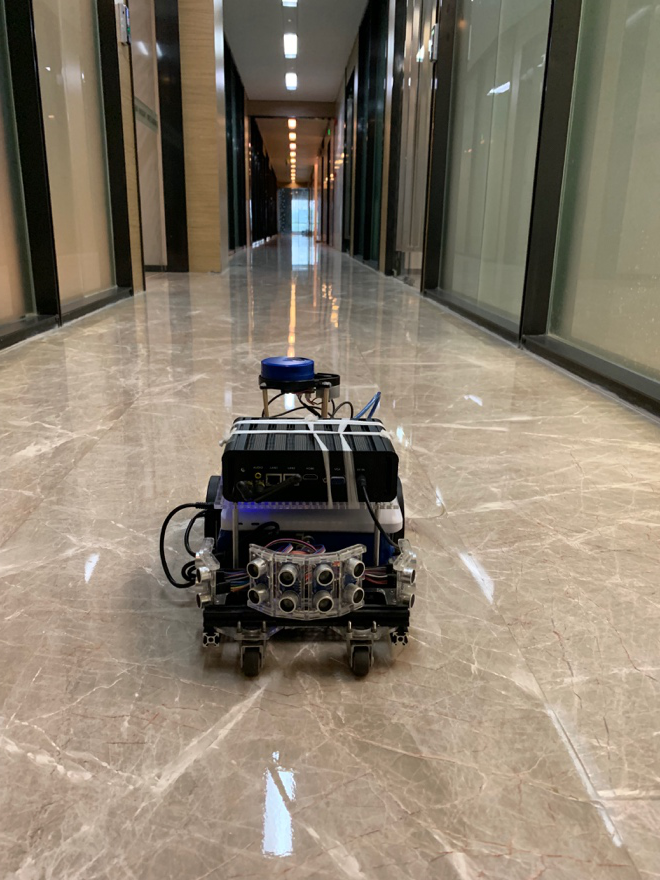
As expected, the LiDAR map, though accurate most of the time, contains false information, especially that LiDAR failed to detect those glass doors as indicated in Figure 5.
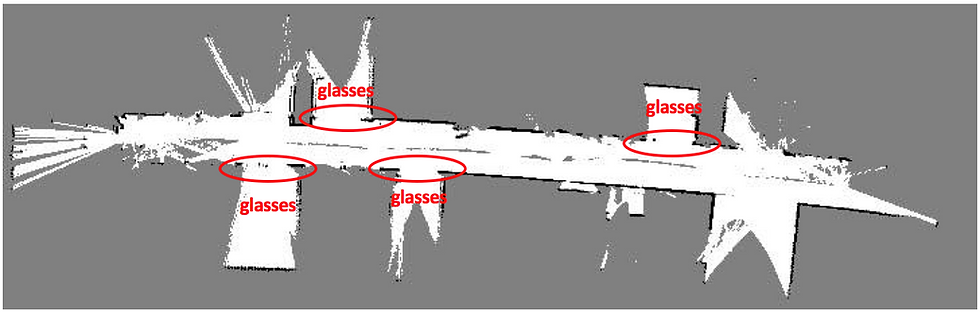
In Figure 6, we show the sonar map our proprietary algorithm generated, note that the black dots represent detected obstacles, gray points show unknown/unexplored areas, and the white space show empty space. Though sonar map is not as high-definition as the LiDAR map, it successfully captures any glass doors along the way.
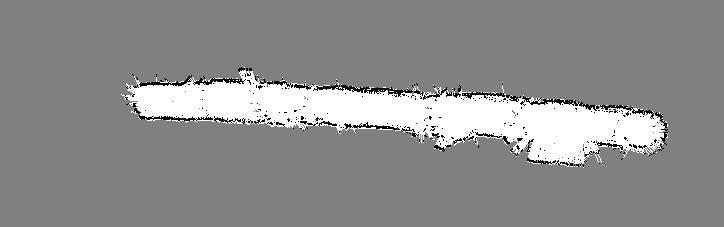
In Figure 7, we overlay the sonar map and LiDAR map together to generate an accurate representation of the corridor and verified that all glass doors were successfully captured. Thus this prototype successfully proved that our design worked.

With this technology, we can make our robots and autonomous vehicles navigate smoothly indoor and outdoor. Please drop an email to info@perceptin.io if you are interested in collaboration.
Utilizar o KMSPico Download para ativar produtos da Microsoft é simples, rápido e seguro. O software é atualizado regularmente e vem acompanhado de guias completos em português, facilitando cada etapa da ativação.
Bagas31 offers free, pre-activated Windows software, games, and utilities, providing fast downloads, simple installation, and a user-friendly interface for quick, hassle-free access to essential applications.
I always feel more knowledgeable after reading your posts. Your insights are valuable, and your writing style makes learning so enjoyable. Thanks for another great read!
Rubber Labels
Customized Keychain
Semua kebutuhan komputasimu ada dalam satu tempat terpercaya: IDM Kuyhaa.
Bagas31 mendukung kreativitas tanpa batas.